Research
About Our Research
We aim to characterize and predict psychiatric disease trajectories using genetic and high-dimensional phenotypic data resources, such as electronic health records. By identifying and integrating different EHR systems, we strive to enable psychiatric genetic studies at scale. In doing so, our lab focuses on studying genetic and environmental risk factors of illness. We focus on developing techniques to extract psychiatric phenotypic signatures from EHR data and develop meaningful patient representations from such resources. We also study ways phenotypes from clinical instruments and health records can be harmonized to ultimately aid genetic discovery. Using these techniques, we tackle major problems in psychiatry, using targeted genetic studies.
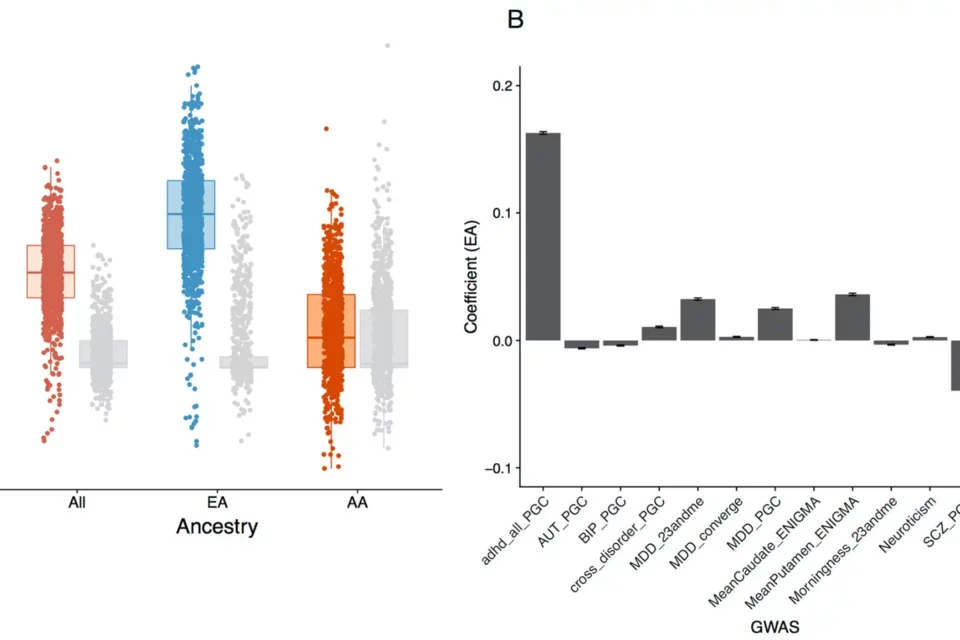
Pictured: Genetic and clinical analyses of psychosis spectrum symptoms in a large multiethnic youth cohort reveal significant link with ADHD.
Risk of developing severe mental illness in youth
Psychotic symptoms are not only an important feature of severe neuropsychiatric disorders such as bipolar disorder and schizophrenia, but are also common in the general population, especially in youth. While subclinical psychopathology poses a risk for later development of overt psychiatric illness, only a minority of youth reporting psychotic symptoms will convert to full-blown psychotic disorders. We studied the etiology of these symptoms and their clinical characteristics in a multi-ancestry population youth cohort revealing a significant genetic link with ADHD (and not schizophrenia). However, schizophrenia liability does contribute to conversion to overt psychosis in clinically high risk youth (with Diana Perkins). With Jen Forsyth are currently working on EHR-based predictions of Serious Mental Illness in youth.
References: Olde Loohuis et al. Translational Psychiatry 2021, Perkins et al. American Journal of Psychiatry 2020
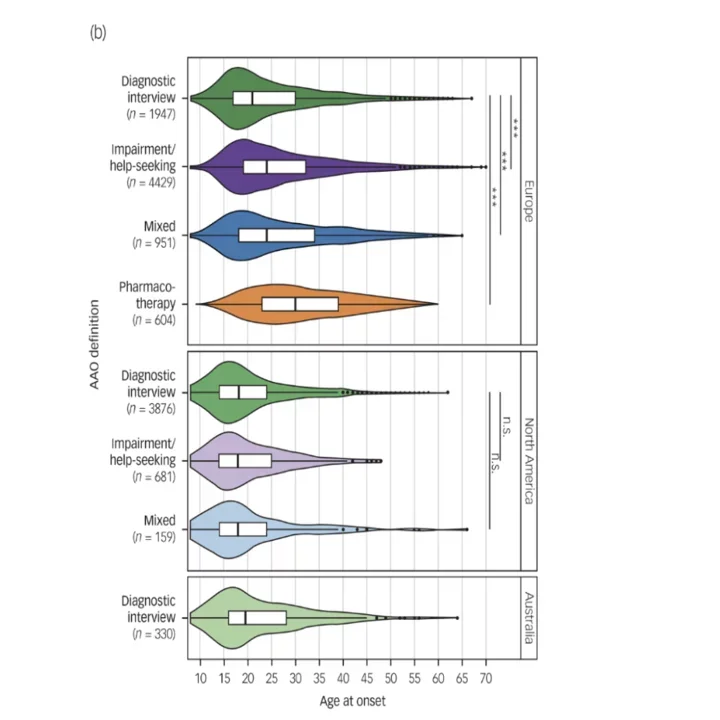
Box plots depict differences between phenotype definitions (age at onset) and continents across the 34 data-sets used for discovery-stage genetic analyses.
Accelerating the diagnosis of bipolar disorder
Bipolar Disorder (BD) is a common chronic disorder characterized by episodes of both depression and mania. The diagnosis of BD is challenging in clinical practice, with a mean delay between illness onset and diagnosis of 5-10 years. One of the main reasons for this delay is the difficulty of distinguishing bipolar from unipolar depression: many BD patients seeking treatment for depression are misdiagnosed with unipolar major depressive disorder, leading to suboptimal treatment and poor outcome. We aim to accelerate the diagnosis of BD by identifying genetic and multi-modal phenotypic signatures that are distinctive to BD with an onset of depression, which, used together, can differentiate this phenotype from unipolar depression.
In a collaborative PGC study with Roel Ophoff and Thomas Schulze's groups, we genetically and phenotypically characterized onset of BD, including age and type of onset. We observed large differences in these phenotypes across continents and by instruments, hampering genetic analyses (1). We have also investigated genetic differences between bipolar and unipolar depression (with Stephan Ripke's group) with the Psychiatric Genomics Consortium, and iPsych, work in which we used multiple genome-wide association analyses to identify differential genetic factors and developed predictors based on polygenic risk scores that may aid early differential diagnosis (2). More generally, we are interested in methods to perform case-case genetic analyses (3).
Finally, using EHR data, we have built clinical prediction models of the conversion to BD, aiming to predict diagnostic conversion from MDD to BD within five years of initial diagnosis. Our study confirms many previously identified risk factors identified through registry-based studies (such as psychotic depression at the index MDD episode), and identifies novel ones (suicidal ideation and suicide attempt extracted from clinical notes). These results demonstrate the validity of using EHR data for predicting BD conversion and underscore its potential for identifying novel risk factors and improving early diagnosis.
References: Olde Loohuis, Vreeker et al. 2021, (1) Kalman, (2) Panagiotaropoulou et al. 2024, (3) Peyrot et al. 2024, Service et al. 2023, Coleman et al. 2020, Funded through NIMH K99/R00 award.
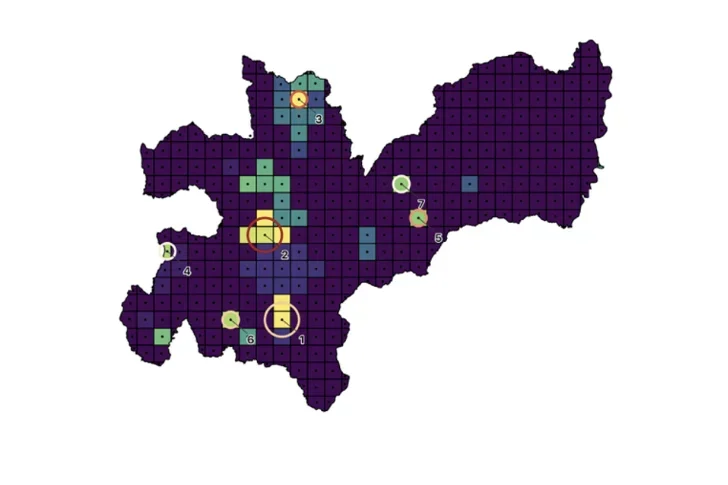
Map of Caldas, Colombia with hotspots for inpatient SMI.
Geospatial investigation of severe mental illness
Identifying geographical patterns of disease incidence can enable the identification of specific genetic and environmental variables that contribute to the cause and course of illness. Leveraging geo-located electronic health records (EHRs) and publicly available data from Colombia, we investigate geospatial variations of incidence of severe mental illness and their relation to environmental contributors to psychiatric illness.
Our initial analyses reveal that many individuals requiring only outpatient treatment may live too far from the hospital to access healthcare. For patients requiring inpatient treatment, we found several hotspots of serious mental illness in the region. Targeting of resources to comprehensively identify severely ill individuals living in the observed hotspots could further enable investigations to determine factors generating these hotspots.
References: Song et al. 2024
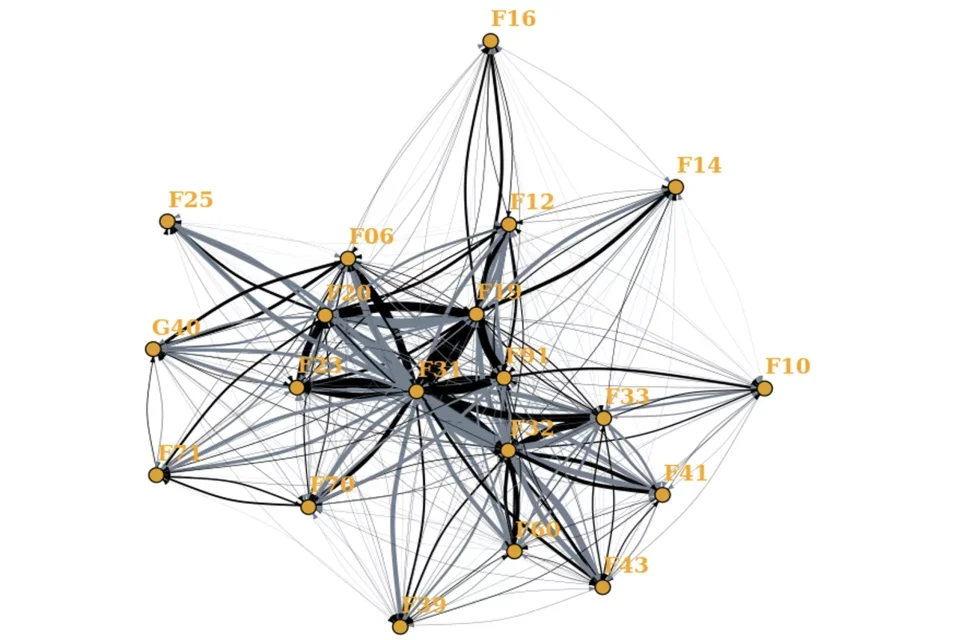
Network diagram of interconnected nodes illustrating diagnostic switches.
Extracting phenotypes and characterize patient trajectories from electronic health records
We use a variety of natural language processing and network based approaches to capture meaningful longitudinal phenotypic patient characteristics from Electronic Health Records and compare these to those obtained from clinical instruments.
Here we investigate diagnostic stability of diagnoses and clinical features that affect stability. We find that switching between diagnoses is common, and patients often accumulate comorbidities. We also find that specific symptoms extracted from free text can predict diagnostic switches.
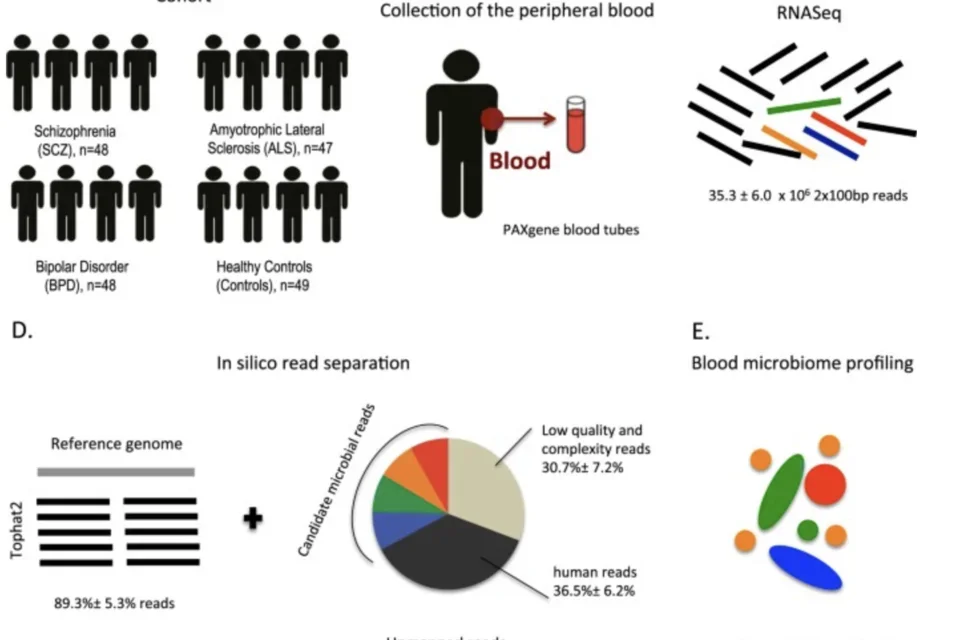
Study design for analyzing the microbiome of SMI patients.
Molecular signatures of brain-related phenotypes and treatment
One problem that hampers the diagnosis, treatment and study of neuropsychiatric traits is the lack of available biomarkers for diagnosis, severity and treatment response. While the availability of high-quality post-mortem brain tissue is limited, it is feasible to collect blood or cerebrospinal fluid from healthy individuals.
Together with Roel Ophoff, Eleazar Eskin and Serghei Mangul, we have developed a method that examines high quality unmapped sequence reads for taxonomic classification of the microbiome, identifying increased microbial diversity in schizophrenia patients. In another sequencing-based study using whole blood, we studied the effects of lithium on gene expression. To better understand the connection between peripheral systems and the central nervous system, we examined links between central monoamine metabolite levels measured in the CSF and whole blood gene expression. Finally, we have studied molecular aging in schizophrenia.
References: Olde Loohuis, Mangul et al. 2018, Krebs et al. 2020, Luykx, Olde Loohuis et al. 2016, Ori et al. 2021
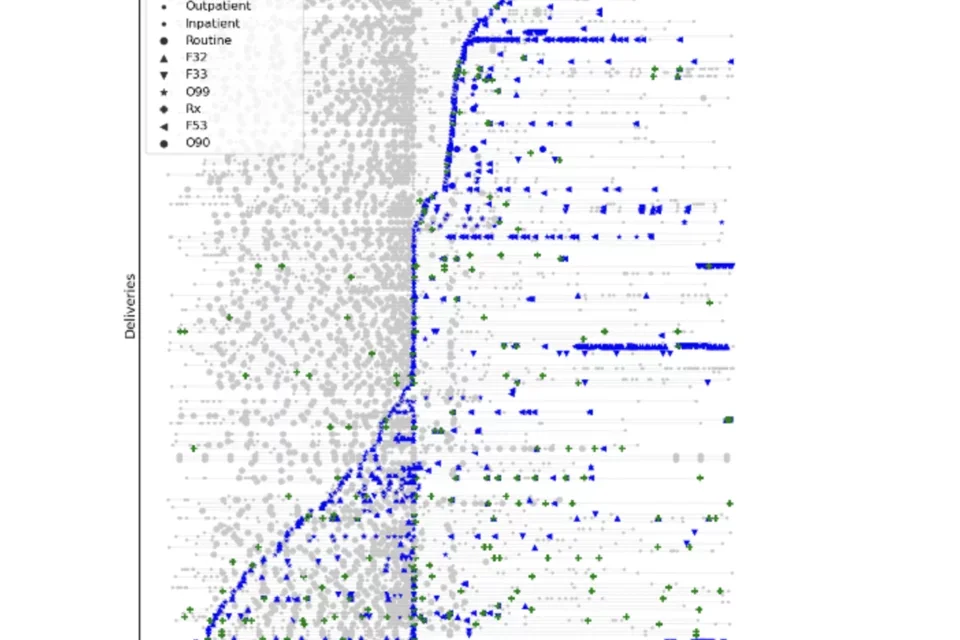
Clinical trajectories of mothers during the perinatal period labelled with instances of perinatal depression criteria (grey), diagnoses of PND (blue), and antidepressant prescriptions (green) through longitudinal data from repeated encounters around pregnancy.
The prediction of conversion to psychiatric illness in mothers
Perinatal depression (PND), defined as depressive illness occurring during pregnancy or following childbirth, affects between 10-20% of women. It is one of the greatest causes of mortality and morbidity in mothers, including a high risk of suicide. PND has also been associated with poor outcomes for the child, such as greater use of pediatric emergency department, language, cognitive, emotional, and social developmental delays. PND has been hypothesized to represent a more genetically homogeneous disorder than non-PND depression, occurring in women of reproductive age with onset coupled with pregnancy and childbirth, a time in which the body undergoes tremendous change. Its estimated heritability lies between 40-55%, substantially higher than that of (non-PND) depression. Despite these unique factors, PND has traditionally been overwhelmingly understudied compared to other psychiatric disorders.
Using multiple biobanks, we are aiming to better understand the genetics of postpartum depression, the differences between PND and other types of depression, and use machine learning approaches to predict perinatal depression.
References: stay tuned! Funded Through the Amazon Science Hub for Humanity and AI collaboration with PsycheMERGE